68%
Reduction
Two third of incidents eliminated
22%
Decrease
In TTR on average.
370+
Hours
Saved each month on average.
The Challenge
The growth of the software usage resulted in an exponential growth of alerts and incidents for the software vendor, who sought a smart monitoring solution to improve the efficiency of the service department in handling these incidents while lowering the total number of false positives and trivial issues to keep both their KPIs and the team motivation high.
Background of the Project
The recent growth in installations base and number of active users had grown the number of alerts and incidents to almost three fold the previous monthly average. A big chunk of these incidents were false positives, mundane alerts like timeouts, or even consequential alert, triggered by a preceding error before the team could resolve it.
The expansion of the team was in discussion, but it would have taken quite some time to train the new employees and thus it would have impacted the productivity of the team on the short term with the addition of the training of new colleagues to their workload.
The option of lowering the monitoring sensitivity posed on the other hand the risk of growing the rate of false negatives and thus impacting user experience.
Thus the best option was to improve the quality of monitoring instead.
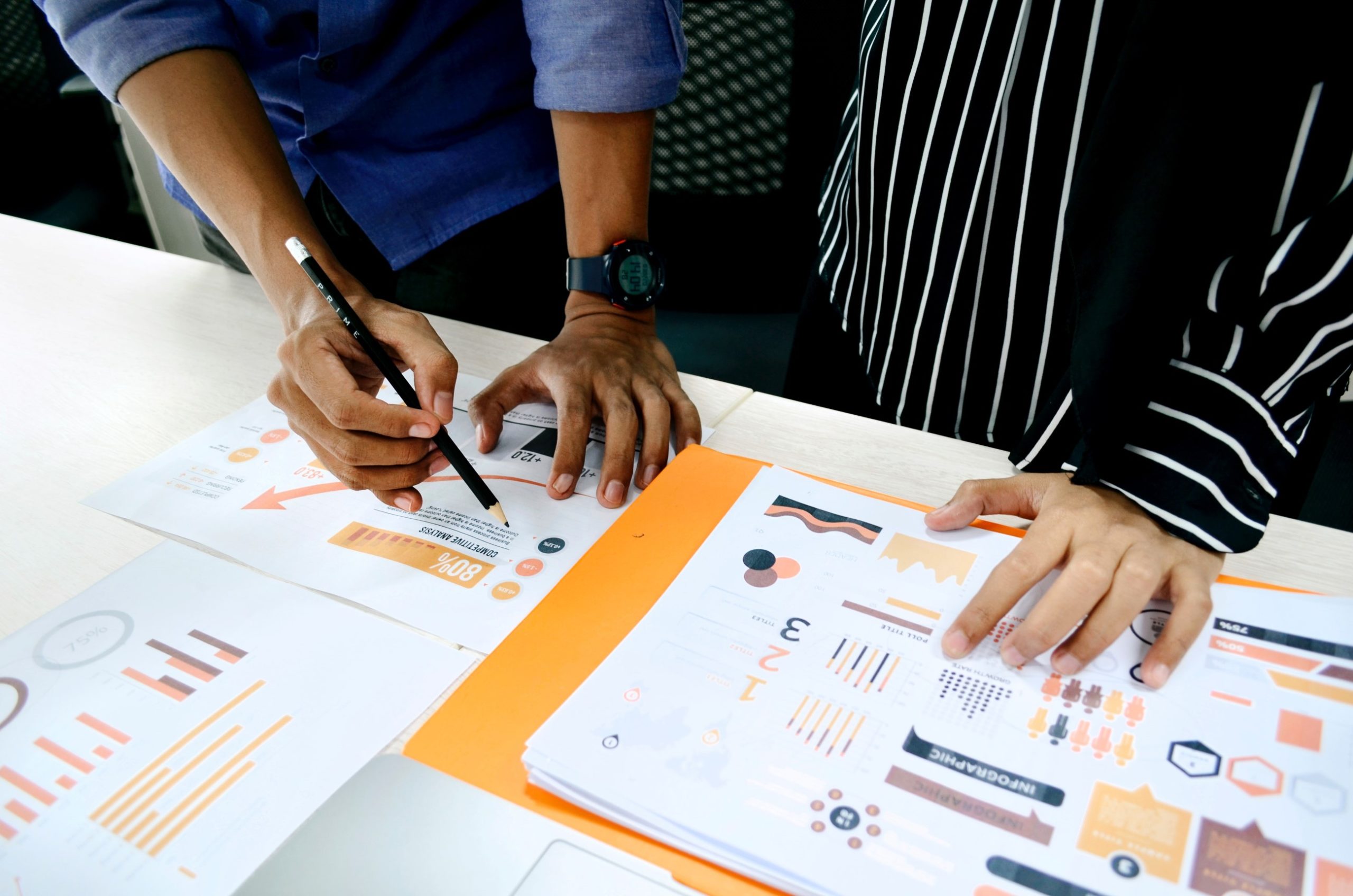
The Process
The project started with the analysis of the current alerts and KPIs in a couple of sessions with the development and operations teams. The target was to create a classification of alerts and identify reoccurring issues the teams felt they were a trivial or mundane. These are errors the teams have faced a few times before, for which a workaround or KEDB entry existed already or alerts that would be repeating false positives, the team just got used to ignore or live with.
Tune down the noise to focus on what really impacted user experience.
From there we started creating custom plugins for the used monitoring tool to enable a dynamic smart handling of repeated alerts. This meant the tool would perform predefined actions for alerts that preceded known errors as soon as it occurs before the monitoring tool would trigger an incident. The added functionalities also enabled a correlation recognition, e.g. consequential errors were recognized. Thus it created a dynamic handling of alerts before the rigid monitoring tool triggered it’s alerts.
The target was to be tune down the “noise” and enable the team to focus on the important incidents, identify real issues that impacted the software quality and the user experience.
Conclusion
The project resulted in the average number of alerts reduced within a few weeks to less than one third of the previous period. The KPIs has improved greatly and with it the team satisfaction, as they could focus again on improving the software quality and user experience, instead of digging through the plethora of trivial alerts trying to meet their target KPIs.
The changed monitoring lead to better insights.
The adapted monitoring helped improve the general understanding of alerts and their relationships. It helped the team recognize early signs of bigger errors and act before they had to react under pressure of reducing down times.
The project lead to better software quality.
This enabled the teams to focus better on the factor that impacted the user experience the most. It also enabled them to see what additional metrics could be added to improve reliability and availability.